All posts by admin
Tennant Creek, Northern Territory, Australia
Tennant Creek NT [BoM ID 015135 (airport), 015087 (post office); joined 1 November 1970] Temperature data from February 1910 to December 2022.
Is homogenisation of Australian temperature data any good?
Dr Bill Johnston, former research scientist, NSW Department of Natural Resources
www.bomwatch.com.au
Protocols, which are essentially a description of the research question (hypothesis) and the means by which it would be addressed, lie at the heart of the scientific method. This report outlines the basis for, and steps involved in undertaking unbiased analysis of trend and change in Australian maximum temperature (Tmax) datasets using Tennant Creek as the case study.
Based on the First Law of Thermodynamics, BomWatch protocols are transparent, objective and replicable and provide a firm baseis for assessing trend and change in maximum temperature datasets and for resolving issues related to the Bureau of Meteorology’s homogenisation of the same data.
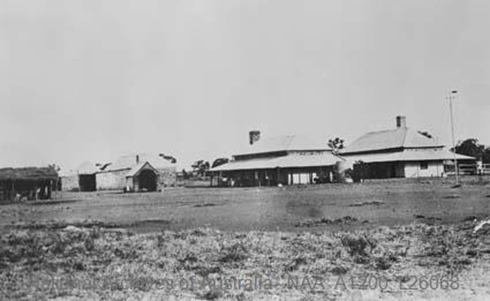
The Tennant Creek Telegraph station in 1872, showing a wind vane and Stevenson screen on the far right of the photograph (National Archives of Australia Item ID: 11774998). As the site was close to buildings, it was probably watered by bucket or watering-can during the seasonal ‘dry’.
Summary
The case study concluded that step-changes related to site changes caused Tmax to increase 1.51oC between 1910 and 2022 independently of rainfall, and that site-changes and rainfall simultaneously explained 73.8% of Tmax variation. Post hoc analysis found no residual trend or change was attributable to any other factor including CO2, coalmining, electricity generation or anything else.
Homogenisation cooled pre-1935 and pre-1963 Tmax to varying extents and achieved trends approaching 0.15oC/decade. Australian Climate Observations Reference Network – Surface Air Temperature V.2.4 also adjusted data higher from 1999 to 2015 by 0.52oC thereby smoothing the effect of post-2013 site changes so they appeared to be due to the climate. However, while trend became more significant and goodness-of-fit improved, variation in Tmax explained by rainfall declined from 46.3% initially, to 16.5% for ACORN-SATv.2.4.
Maps, plans, aerial photographs and satellite images show unequivocally that trend in Tmax data was mostly due to spraying out the grass and replacing the former 230-litre Stevenson screen in 2012, and installing a wind-profiler array within 45m of the screen before March 2013.
Bomwatch protocols
BomWatch protocols comprise four elements, namely:
· The overall relationship between Tmax and rainfall partitions total variation into that due to rainfall, and the residual non-rainfall part. Linear regression also derives the overall Tmax/rainfall coefficient, and significance (P) and goodness of fit (R2adj) statistics that indicate conformity with the First Law of Thermodynamics.
· Homogeneity analysis of rescaled residuals identifies non-climate impacts on data, which are categorised as step-change or (Sh)ift scenarios
· Segment-by-segment analysis with rainfall detects outliers, lack of fit, and other potential problems, and,
· Categorical multiple linear regression (and interaction analysis) finalises and verifies outcomes.
Segmented trend and graphical analysis confirm and verify that relationships are linear, residuals are normally distributed, independent, with constant variance, and that they are timewise homogeneous.
Based on the First Law Theorem that maximum temperature depends on rainfall, BomWatch protocols provide an unequivocable basis for understanding the effect of non-climate impacts on data, and for objectively assessing the BoM’s homogenisation methods.
Click here to download the full paper with photos, graphs and data
Bill Johnston
6 February 2024
Timber Creek, Northern Territory, Australia
Is homogenisation of Australian temperature data any good?
Is homogenisation of Australian temperature data any good?
Part 7b. Timber Creek, Northern Territory, Australia
Bureau of Meteorology ID 14850, Latitude -15.6614 Longitude 130.4808.
Dr Bill Johnston
scientist@bomwatch.com.au
Summary
Data quality is poor. The use of faulty data that are not homogeneous, to adjust faults in ACORN-SAT data is unscientific and likely to result in biased outcomes. As the ACORN-SAT project does not ensure comparator datasets are homogeneous, it is deeply flawed and should be abandoned. Read on …
The quality of Tmax data for Timber Creek is exceptionally poor. Its use for adjusting faults in ACORN-SAT data is highly questionable. Data are affected by missing daily data, poor site-control and the probable replacement of a 230-litre Stevenson screen with a 60-litre one around 1996. Although data lack precision, accounting for rainfall and the up-step in 1996 left no residual variation attributable to CO2, coalmining, electricity generation or anything else.
Background
The isolated town of Timber Creek is located beside the Victoria River, on the Victoria Highway, 600km south of Darwin and 280m southwest of Katherine in the Northern Territory (Figure 1). The Victoria River flows west to the Joseph Bonaparte Gulf close to the Western Australia – Northern Territory border. Daily maximum temperature (Tmax) data is available from the Bureau of Meteorology (BoM) from 23 January 1961, to when the station closed on 5 September 2014.
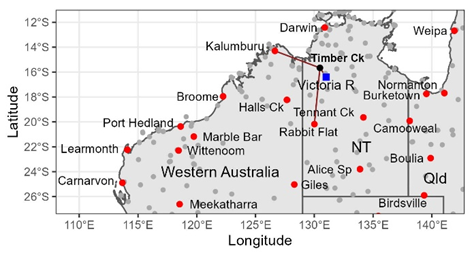
Figure 1. Timber Creek and other weather stations having more than 10-years of records (grey points), and Victoria River Downs (blue) and other ACORN-SAT sites in northern Australia (red buttons).
Tmax data for Timber Creek was used to adjust ACORN-SAT sites at Victoria River Downs, Kalumburu, and Rabbit Flat (Table 1). So, is Tmax data for Timber Creek any good?
Table 1. ACORN-SAT sites adjusted at the dates indicated using Tmax data for Timber Creek.
ACORN-SAT site: (Distance to Timber Ck.) | Vic. River Downs (ID 14825) (106km) | Kalumburu (ID 1019) (434km) | Rabbit Flat (ID 15666) (509km) |
Adjust dates AcV1 (2009) | 1 Aug 1987 | 1 Jan 1986 23 Aug 1991 | Nil |
Adjust dates AcV2.1 (2020) | Nil | 1 Jan 1986 23 Aug 1991 | 1 March 1997 |
Adjust Dates AcV2.3 (2023) | Nil | 1 Jan 1986 23 Aug 1991 | 1 March 1997 |
Methods
Daily temperature and monthly rainfall were downloaded from the Bureau of Meteorology, Climate Data Online facility (Climate Data Online – Map search (bom.gov.au)). Monthly rainfall was infilled as necessary using data for the nearest available site and flagged for reference. Data were summarised into an annual dataset (Timber Creek.xlsx) and analysed using the same BomWatch protocols described previously in the Parafield case study1 and subsequent reports, including for Victoria River Downs2 of which this report is a subset.
Only maximum temperature (Tmax) data were analysed.
Results
Missing daily observations may have affected mean annual Tmax in 1987, 2008 and 2013 (N <346 observations/year); however, influence plots did not suggest those years were outliers. Outlier years which were identified (but also not omitted) were 1998, 2005 and 2013. Observations appeared to become lackadaisical after 2006. No other attributes of the data were remarkable.
Rainfall, which is the deterministic portion of the Tmax signal explained 27.0% of Tmax variation (Figure 2(a)), which is low relative to the BomWatch benchmark of R2adj = 0.50. Either data quality is exceedingly poor, or an influential variable has not been accounted for by the naïve Tmax ~ rainfall case (Table 1(i)).
(Note: R2adj calculated by the statistical package R, adjusts variation explained for the number of terms in the linear model, as well as for the number of observations3. It is therefore more robust (less biased) than unadjusted R2 calculated by spreadsheet programs such as Excel.)
Figure 2. Composite analysis of Timber Creek Tmax.
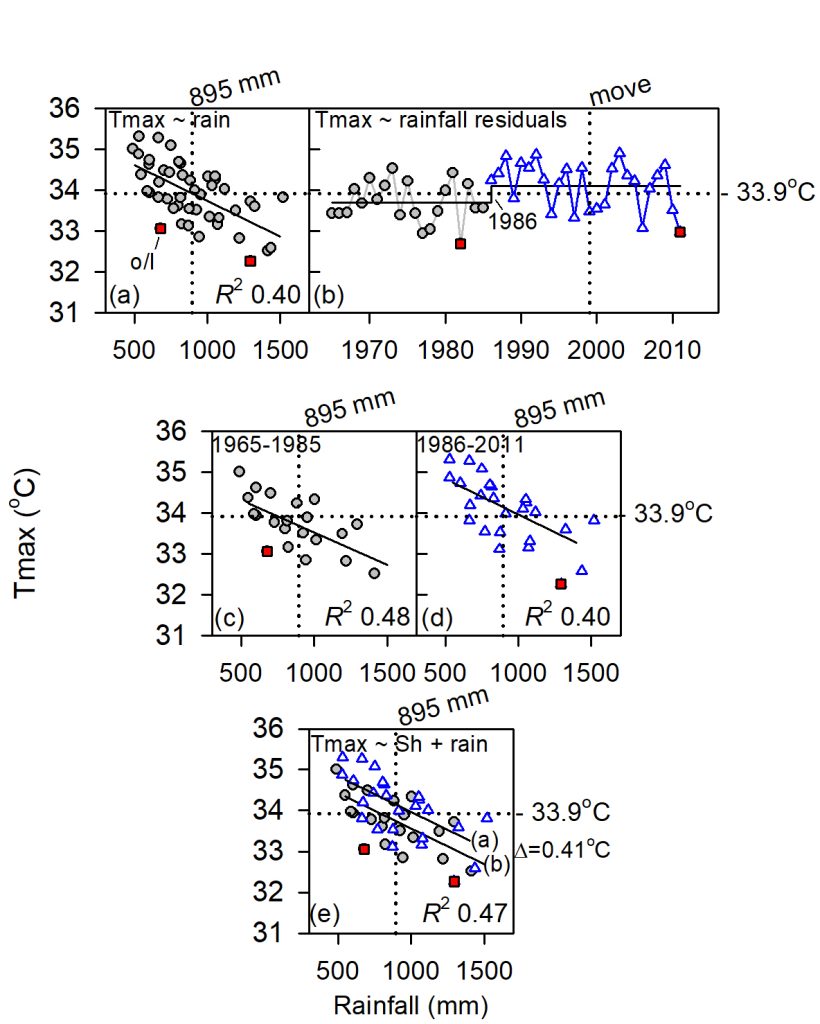
Inhomogeneities in rescaled Tmax ~ rainfall residuals were evaluated using STARS, which objectively tests whether the mean of subsequent values is significantly different (P<0.05) to that before, using a running t-test of the difference.
Indicated by the horizontal line in Figure 2(b), STARS detected an up-step of 0.47oC in 1996 (P = 0.003). Segments defined by the step-change were examined separately in Figure 2(c) and Figure 2(d) and Table 1(ii).
Categorical multiple linear regression (Table 1(iii)) showed rainfall reduces Tmax 0.175oC/100mm, and that segmented regressions were offset by a rainfall-adjusted difference of 0.58oC (Figure 2(e)), which is within range of that detected by STARS.
Post hoc tests (Table 1(iv)), confirmed that data consisted of two non-trending segments interrupted by the step-change in 1996.
Although data lack precision (R2adj <0.50, Table 1(iii)), accounting for rainfall and the step change in 1996, left no residual variation attributable to CO2, coalmining, electricity generation or anything else.
Table 1. Statistical summary. RSS refers to residual sum of squares. Partial R-square (R2partial) estimates the proportion of variation explained by the Sh(ift)factor that is not explained by rainfall alone (calculated as: [(RSSfull – RSSrain)/ RSSfull)*100].
Model | Coef. (oC/100mm) | P | R2adj | Segment | RainAdj (SE) (oC)(1) | RSS (R2partial) | |
(i) Tmax ~ rain (all) | -0.151 | 0.001 | 0.270 | 7.64 | |||
(ii) Tmax ~ rain(2) 1961-1995 1996-2013 | -0.192 -0.240 | 0.005 0.001 | 0.420 0.464 | ||||
(iii) Tmax ~ Shres + rain(2) | -0.175 | <0.001 | 0.473 | 1961-1995 1996-2013 Delta(1 vs 2) | 34.6(a) (0.120) 35.2(b) (0.109) 0.58 (0.173) | 5.56 (37.5%) | Interaction Tmax ~ Shres * rain ns |
(iv) Tmax ~ Year(2) 1961-2013 1961-1995 1996-2013 | (oC/decade) 0.106 0.617 -0.196 | 0.325 0.329 0.528 | ns ns ns | ||||
(1) Letters in parenthesis indicate differences between means (2) No outliers |
Discussion
Relationships between Tmax and rainfall (Table 1) show shows the quality of Tmax data for Timber Creek is exceptionally poor, which raises the question why would it be used to adjust ACORN-SAT sites at Victoria River Downs, Kalumburu, and Rabbit Flat? Furthermore, the step-change in 1996 is likely due to the former 230-litre Stevenson screen being replaced by a 60-litre one during the previous year.
Despite problems with watering and missing observations, Victoria River Downs is one of only several closed or open sites within 200km with reasonable runs of Tmax data (Kununurra town and airport and Kimberley Research Station being others). Although Timber Creek closed in September 2014, it seems from available Google Earth Pro satellite images the site was watered (Figure 3).
Figure 3. The satellite image of the alleged Timber Creek weather station site in August 2022 at Latitude -15.6614o, Longitude 130.4808o. However, the site looks nothing like the site diagram shown in site-summary metadata. There is no sign of the ‘chook shed’, while the ‘house’, appears to be the Timber Creek Travellers Rest Motel and caravan park. Successive images also show the grass surrounding the site is regularly watered. Either the coordinates provided in metadata are incorrect or the diagram is inaccurate.
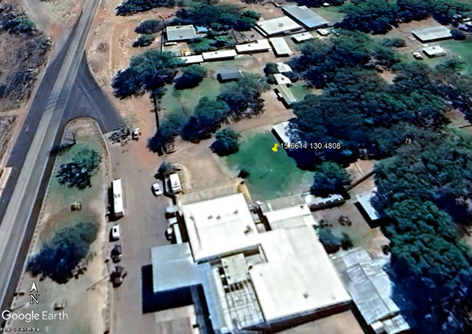
The problem is that site-summary metadata is faulty.
Close inspection of properties around the town, located the Stevenson screen inside a fenced area that matched the site diagram, at Latitude -15.6600o, Longitude 130.4819o, approximately 190m NE of the location shown in Figure 3.
While regular manual observations may have ceased, the most recent satellite image (August 2023) shows the Stevenson screen is still located within the enclosure. Images from August 2002, show land-use changes, possibly irrigation and the use of herbicide between seasons and years contributed variation that impacted observations, and therefore that lack of site control was the main factor affecting the quality of Tmax data.
Given the paucity of nearby sites, and the lack of useful long-term data, Timber Creek is not such a big overcrowded place that the BoM could not have installed an automatic weather station on vacant land somewhere in the town where site conditions were consistent with the local climate – high monsoonal rainfall from November to March (summer) and low rainfall during the ‘dry’. Unreliable metadata, including routinely failing to document when Stevenson screens are replaced, and lack of site control is a major problem across Australia’s weather station network.
Conclusion
It is concluded that the quality of Tmax data for Timber Creek is exceptionally poor and its use for adjusting faults in ACORN-SAT data at Victoria River Downs, Kalumburu and Rabbit Flat is highly questionable. Data are affected by missing daily data, poor site-control and the probable replacement of a 230-litre Stevenson screen with a 60-litre one around 1996. Although data lack precision, accounting for rainfall and the step change in 1996 left no residual variation attributable to CO2, coalmining, electricity generation or anything else.
Bill Johnston
18 February 2024
Preferred citation:
Johnston, Bill 2024. Is homogenisation of Australian temperature data any good? Part 7b. Timber Creek, Northern Territory, Australia http://www.bomwatch.com.au/ 4 pp.
Disclaimer
Unethical scientific practices including the homogenisation of data to support political narratives undermines trust in science. While the persons mentioned or critiqued may be upstanding citizens, which is not in question, the problem lies with their approach to data, use of poor data or their portrayal of data in their cited and referenceable publications as representing facts that are unsubstantiated, statistically questionable or not true. The debate is therefore a scientific one, not a personal one.
Acknowledgements
David Mason-Jones is gratefully acknowledged for providing invaluable editorial assistance. Research
Larimah, Northern Territory, Australia
Is homogenisation of Australian temperature data any good?
Part 7a. Larrimah, Northern Territory, Australia
Bureau of Meteorology ID 014612. Located 430 km SE of Darwin at Latitude -15.5748 Longitude 133.2137. Maximum temperature data from 1 January 1965 to 29 June 2012.
Dr Bill Johnston[1]
Data quality is poor. The use of faulty data that are not homogeneous, to adjust faults in ACORN-SAT data is unscientific and likely to result in biased outcomes. As the ACORN-SAT project does not ensure comparator datasets are homogeneous, it is deeply flawed and should be abandoned. Read on …
Summary
While data quality is poor, accounting for rainfall and the step-change simultaneously using multiple linear regression left no additional trend or change that could be attributed to the climate, CO2, coalmining or anything else.
1. Background
Situated on the Stuart Highway 430 km southeast of Darwin (Figure 1) maximum temperature (Tmax) observations at Larrimah ceased on 29 June 2012. However, Larrimah Tmax was used by ACORN-SAT (the Australian Climate Observations Reference Network – Surface Air Temperature project) to homogenise Tmax data at Victoria River Downs (1/1/1976 and 1/8/1987, AcV1 and 1/1/2007, AcV2.x) and Burketown airport (1/1/2002, AcV1 and 1/1/1986, AcV2.x).
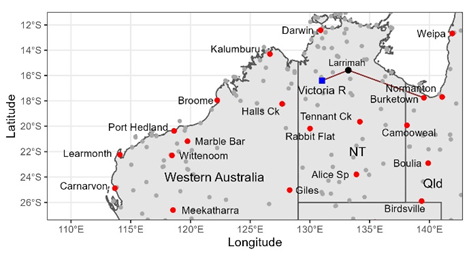
Figure 1. Larimah Tmax was used to homogenise Tmax data for Victoria River Downs (256km away), and Burketown airport (762 km distant). ACORN-SAT sites are indicated by red buttons, and sites having more than 10-years of data, by grey circles.
This raises the question: is Tmax data for Larrimah any good?
2. Methods
Daily temperature and monthly rainfall were downloaded from the Bureau of Meteorology, Climate Data Online facility (Climate Data Online – Map search (bom.gov.au)). Data were summarised into an annual dataset and analysed using the same BomWatch protocols described previously in the Parafield case study[2] and subsequent reports, including for Victoria River Downs[3] of which this report is a subset.
Only maximum temperature (Tmax) data are used in the study.
3. Results
Ignoring outliers (red squares in Figure 2(a)), rainfall, which is the deterministic portion of the Tmax signal explains 39.5% of Tmax variation (Radj = 0.395). As this is less than the R2adj BomWatch benchmark of 0.50, either data quality is exceedingly poor, or an influential variable has not been accounted for by the naïve Tmax ~ rainfall case (Table 1(i)).
(Note: R2adj calculated by the statistical package R, adjusts variation explained for the number of terms in the linear model, as well as for the number of observations[4]. It is therefore more robust (less biased) than unadjusted R2 calculated by spreadsheet programs such as Excel.)
Rescaled so values are comparable, Tmax ~ rainfall residuals were evaluated for inhomogeneities using STARS, which objectively tests whether the mean of subsequent data is significantly different (P<0.05) to that before, using a t-test of the difference. Indicated by the horizontal line in Figure 2(b), STARS detected an up-step of 0.41oC in 1986 (P = 0.015). Segments defined by the step-change were examined separately in Figure 2(c) and Figure 2(d).
The spread of points about each line, and influence plots (not shown) confirmed that data for 1982 and 2011 were likely outliers. Also, as R2adj was <0.50 data quality was generally poor.
Categorical multiple linear regression of the form Tmax ~ Sh(ift)factor + Rainfall showed rainfall reduces Tmax 0.174oC/100mm, and that segmented regressions were offset by a rainfall adjusted difference of 0.41oC (Figure 2(e)), which is the same as that detected by STARS. Post hoc tests confirmed that data consisted of two non-trending segments interrupted by the step-change in 1986 (Table 1(iv)).
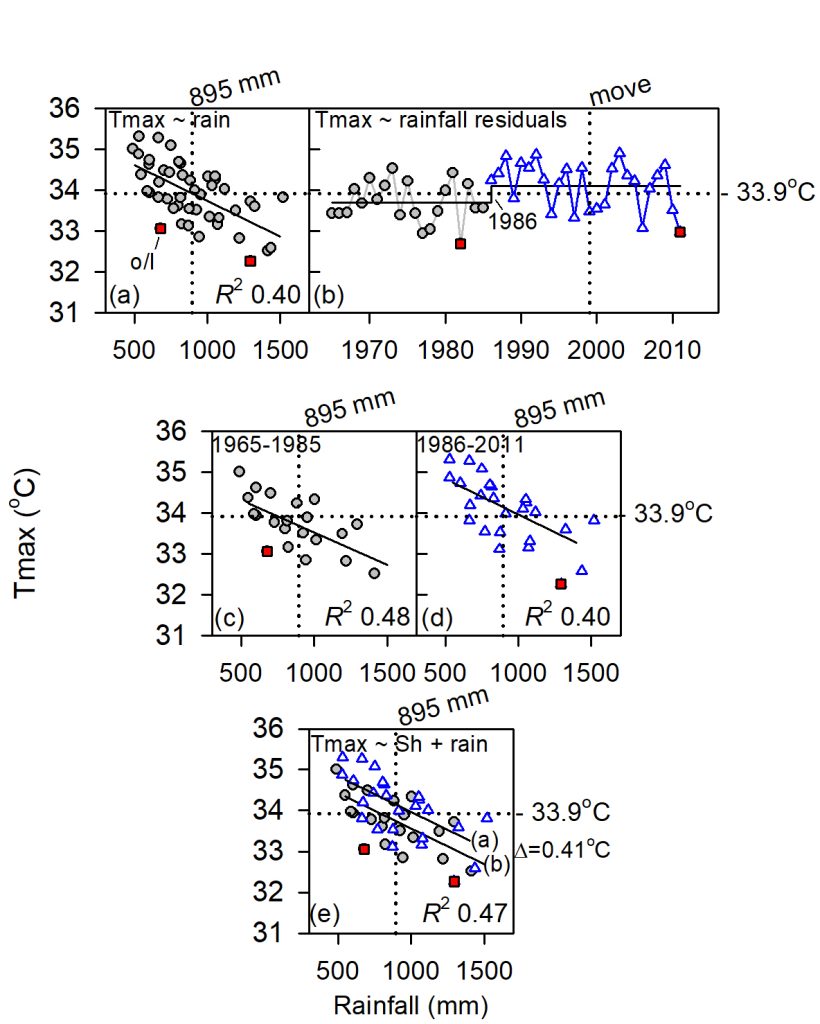
Figure 2. Composite analysis of Larrimah Tmax. A statistical summary for each phase of the analysis is provide in Table 1.
4. Discussion and conclusions
Diagrams in site-summary metadata for 1 September 1964 and 7 December 1968, show the site was originally at the rear of the post office on the western side of the Stuart Highway. However, by 8 June 1992 it had moved to the disused WWII rail terminus of the North Australian Railway, which ceased operations and closed in February 1981.
While the alleged move within the confines of the terminus in November 1998 was not influential, the 1986 step-change was probably due to relocating the site there and replacing the original 230-litre Stevenson screen with a 60-litre one. It seems the move to the trucking-yard, allegedly in 1998, was erroneously reported.
Google Earth Pro satellite images from October 2004 show surrounds of the trucking-yard site were generally bereft of ground cover, which would be sufficient to cause Tmax data to be warmer than at the previous site behind the post office.
Although categorical multiple liner regression confirmed that the up-step in the mean occurred in 1986 (Table 1(iii)), low R2adj and apparent overlap in scatter between the series (Figure 2(e)) show data were of exceptionally poor quality, particularly after 1986. This may have been due to lackadaisical observing practices, including excessive numbers of missing data/year. (Fewer than 340 observations were noted in 1970, 1975 and 1976, and from 1994 to 1998). Although given its role in ACORN-SAT and that the site is isolated, it is surprising that the BoM did not install an automatic weather station at Larrimah. The current site probably closed due to a lack of local interest in making observations and undertaking maintenance.
It was concluded that while data quality is poor, accounting for rainfall and the step-change simultaneously using categorical multiple linear regression, left no additional trend or change that could be attributed to the climate, CO2, coalmining or anything else.
Table 1. Statistical summary. RSS refers to residual sum of squares. Partial R-square (R2partial) estimates the proportion of variation explained by the Sh(ift)factor that is not explained by rainfall alone (calculated as: [(RSSfull – RSSrain)/ RSSfull)*100].
Model | Coef. (oC/100mm) | P | R2adj | Segment | RainAdj (SE) (oC)(1) | RSS (R2partial) | |
(i) Tmax ~ rain (all) Not 1982, 2011(2) | -0.176 -0.171 | <0.001 <0.001 | 0.379 0.395 | 10.649 | |||
(ii) Tmax ~ rain(2) 1965-1985 1986-2011 | -0.175 -0.175 | <0.001 0.004 | 0.482 0.400 | ||||
(iii) Tmax ~ Shres + rain(2) | -0.175 | <0.001 | 0.473 | 1965-1985 1986-2011 Delta(1 vs 2) | 33.7(a) (0.113) 34.2(b) (0.101) 0.41 (0.151) | 12.507 (14.9%) | Interaction Tmax ~ Shres * rain ns |
(iv) Tmax ~ Year(2) 1965-2011 1965-1985 1986-2011 | (oC/decade) 0.028 0.240 -0.426(3) | 0.720 0.329 0.025 | ns ns 0.165 | ||||
(1) Letters in parenthesis indicate differences between means (2) Outliers omitted from the analysis (3) Affected by a single extreme value |
Bill Johnston
17 February 2024
Click here for the full Larrimah data
Johnston, Bill 2024. Is homogenisation of Australian temperature data any good? Part 7a. Larrimah, Northern Territory, Australia http://www.bomwatch.com.au/ 3 pp.
Disclaimer
Unethical scientific practices including the homogenisation of data to support political narratives undermines trust in science. While the persons mentioned or critiqued may be upstanding citizens, which is not in question, the problem lies with their approach to data, use of poor data or their portrayal of data in their cited and referenceable publications as representing facts that are unsubstantiated, statistically questionable or not true. The debate is therefore a scientific one, not a personal one.
Acknowledgements
David Mason-Jones is gratefully acknowledged for providing invaluable editorial assistance. Research includes intellectual property that is copyright (©).
[1] Former NSW Department of Natural Resources research scientist and weather observer.
[2] Parafield Ref Welcome to homogenisation – arguably the greatest scam of 20th century science (bomwatch.com.au)
[3] Victoria River Downs Ref VictoriaRiverDowns-16-Feb-2024-1.pdf (bomwatch.com.au)
Victoria River Downs Northern Territory Australia
Is homogenization of Australian weather data any good?
Dr Bill Johnston[1]
Situated 443 km south of Darwin, 410 km NE of Halls Creek and 433 km north of Rabbit Flat in the Kimberley Region of the Northern Territory, the iconic Victoria River Downs Station was once the largest pastoral holding in the world. The station homestead beside the Ord River (Figure 1), is the location of an Australian Climate Observations Reference Network – Surface Air Temperature dataset (ACORN-SAT) weather station, one of 112 such sites used to monitor climate warming in Australia. Due to the sparseness of the Bureau of Meteorology’s (BoM) network in northern Australia, data for Victoria River Downs (BoM ID 14825) is weighted by ACORN-SAT to be representative of some 3.3% of Australia’s land area.
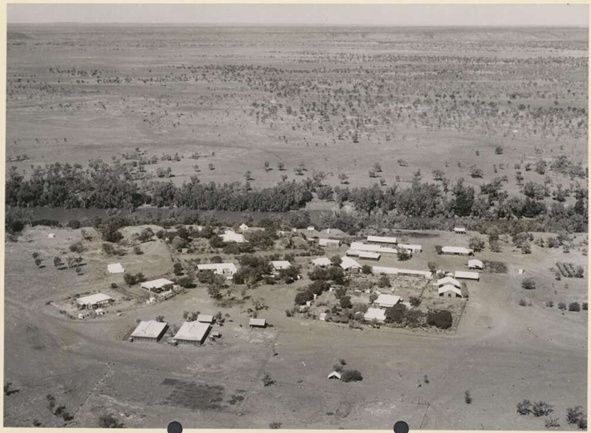
Figure 1. The Victoria River Downs homestead and outbuildings south of the Ord River in the Kimberley Region of the Northern Territory in 1954 (National Library of Australia, copyright expired: https://nla.gov.au:443/tarkine/nla.obj-137684286).
From when observations commenced in 1965 until about 1979 data were plagued by runs of missing observations, or low data counts per month. Observations were also mostly reported in whole and ½oC from 1975 to 1982. The frequency of whole degrees was higher than other decimal-fractions from when the automatic weather station (AWS) was installed in May 1997 until about 2006. Site-surrounds and the lawn beyond were also irregularly watered. Maximum temperature data (Tmax) could not therefore be judged as high-quality. As data were unavailable from June to September 1973 (N=210), 1973 was omitted from the analysis.
The overall Tmax trend of 0.125oC/decade (P = 0.055) was spuriously due to an abrupt Tmax up-step of 1.12oC in 2013, which was not related to a change in the weather or climate. Accounting for that and the effect of rainfall on observations, left no additional signals that could be attributed to CO2, coalmining, electricity generation or anything else.
The change may have been due to cessation of watering (which was said to have ceased in 2007), replacement of a former 230-litre Stevenson screen with a 60-litre one, or replacement of a wooden 60-litre screen with a plastic one. According to site-summary metadata the Tmax thermometer was removed in July 2012 (thus backup manual observations ceased), but curiously, it was re-installed in March 2016 and replaced again in September 2019. Like many ACORN-SAT sites, metadata (data about the data) is vague and unreliable and not a basis for correcting data for site-change effects.
Consistent with the First Law of Thermodynamics, analysis of trend and change was undertaken using BomWatch protocols that are transparent, objective, and replicable, and cannot be ‘fiddled’ to achieve pre-determined outcomes.
With the First Law onside, nothing can possibly go wrong.
Tmax depends on rainfall such that the drier it is the hotter it gets. If the relationship between Tmax and rainfall is not significant, weak or positive, something is wrong with the data, not the First Law Theorem. Further, if variation explained (R2adj) is less than a benchmark of 0.50 (or 50%) data may either embed a ‘missing variable’ (one that is not explained by the naïve Tmax ~ rainfall case), or the quality of data is arguably too poor for determining trend and change in the climate.
Site related inhomogeneities occur in parallel with observations and are therefore confounded with the Tmax signal. However, as the Tmax ~ rainfall relationship implicitly accounts for the rainfall effect, non-rainfall residuals embed all other sources of variation, including underlying systematic changes related to site-changes. The second step in the Protocol investigates residuals for significant shifts or step-changes in re-scaled residuals indicative of such factors. Importantly, changepoints are detected objectively and cannot be specified in advance.
Identified using a factor variable, final-round analysis verifies that segmented responses to rainfall are the same (slopes are parallel), and that rainfall-adjusted segment means are different (that individual relationships are offset). Each of the three BomWatch protocol steps is transparent, objective and replicable, and supported by subsidiary investigations including analysis of residuals and post hoc tests.
Homogenisation is near the limit of plausibility
The same protocols used to analyse Tmax were used to evaluate homogenisation of the same data by ACORN-SAT.
Three iterations of ACORN-SAT applied adjustments at different times such that past-data were cooled and/or warmed to varying extents toward the present, which is a trick that affects trend. However, while adjustments may stabilise and improve statistical significance (and possibly moderate data quality problems), segmented relationships with rainfall become less clear-cut and less precise overall. Lack of statistical control and the absence of post hoc evaluations is a major weakness of ACORN-SAT.
The political narrative supported by ACORN-SAT will eventually be shown to be false, either as more ACORN-SAT sites are analysed using rigorous BomWatch protocols; or, as time passes and BoM scientists run out of options for making adjustments that seem plausible; or, adjustments cause fundamental Tmax ~ rainfall relationships to break-down. The question then arises: just how many more analyses are needed? or, how many years need to pass, before ACORN-SAT is shown irrevocably to be too unsound, unscientific and unbelievable to continue? As the ACORN-SAT project is unsalvageable, for the sake of those involved it should be abandoned without exception, in its entirety.
Implications
The practical implication is that most messaging related to climate warming in Australia, including the indoctrination of vulnerable schoolchildren and young adults, and State of the Climate reports published by CSIRO, is demonstrably fake. The whole warming agenda has been made-up and carried forward since the call went-out from the World Meteorological Organisation in about 1989 to find and supply data that supported future Intergovernmental Panel on Climate Change (IPCC) reports. Temperature data homogenisation resulted from that.
The scientific implication is that along with models used to predict future climates, scores, possibly thousands of scientific papers and reports that depend on the warming narrative, are worthless.
Studies related to the effect of ‘a warming world’ on health, on agriculture, tourism, urban planning, the Murray-Darling Basin, the Great Barrier Reef, urban water supplies, species extinctions, and for converse reasons, on mining and resource use, are based on a premise that has been fabricated by consensus from the beginning.
The political implication is that the billions of dollars that have been spent on, or are intended to be spent, in order to limit warming to the mythical value of 1.5oC sometime in the future, is entirely wasted. With the national debit spiralling out of control, crippling electricity prices, subsidies and carbon taxes flowing from diminishing numbers of primary producers and workers to the elites, will eventually cripple Australia’s ability to remain sovereign, democratic and free.
As The Science is underpinned by data that has been fabricated to support it, and as the manipulations of past-data will become increasingly implausible going forward, the whole edifice must eventually collapse. Collapse will probably occur within a decade, possibly sooner than 2030.
Finally, as Tmax depends on rainfall, which in Australia is stochastic (unpredictable) and episodic (occurs in episodes), without knowing rainfall in advance, it is impossible to predict the trajectory of Tmax into the future.
Dr Bill Johnston
15 February 2024
Preferred citation:
Johnston, Bill 2024. Is homogenisation of Australian temperature data any good? Part 7. Victoria River Downs, Northern Territory, Australia http://www.bomwatch.com.au/ 15 pp.
Click here to download the full paper with photos, graphs and data
Click here for the full Victoria River data
[1] Former NSW Department of Natural Resources research scientist and weather observer.
Analysis of parallel temperature data using t-tests
Part 2. Brisbane Airport
Dr Bill Johnston
Using paired and un-paired t-tests to compare long timeseries of data observed in parallel by instruments housed in the same or different Stevenson screens at one site, or in screens located at different sites, is problematic. Part of the problem is that both tests assume that the air being monitored is the control variable. That air inside the screen is spatially and temporally homogeneous, which for a changeable, turbulent medium is not the case.
Irrespective of whether data are measured on the same day, paired t-tests require the same parcels of air to be monitored by both instruments 100% of the time. As instruments co-located in the same Stevenson screen are in different positions their data cannot be considered ‘paired’ in the sense required by the test. Likewise for instruments in separate screens, and especially if temperature at one site is compared with daily values measured some distance away at another.
As paired t-tests ascribe all variation to subjects (the instruments), and none to the response variable (the air) test outcomes are seriously biased compared to un-paired tests, where variation is ascribed more generally to both the subjects and the response.
The paired t-test compares the mean of the differences between subjects with zero, whereas the un-paired test compares subject means with each other. If the tests find a low probability (P) that that the mean difference is zero, or that subject means are the same, typically less than (P<) 0.05, 5% or 1 in 20, it can be concluded that subjects differ in their response (i.e., the difference is significant). Should probability be less than 0.01 (P<0.01 = 1% or 1 in 100) the between-subject difference is highly significant. However, significance itself does not ensure that the size of difference is meaningful in the overall scheme of things.
Assumptions
All statistical tests are based on underlying assumptions that ensure results are trustworthy and unbiased. The main assumption for is that differences in the case of paired tests, and for unpaired tests, data sequenced within treatment groups are independent meaning that data for one time are not serially correlated with data for other times. As timeseries embed seasonal cycles and in some cases trends, steps must be taken to identify and mitigate autocorrelation prior to undertaking either test.
A second, but less important assumption for large datasets, is that data are distributed within a bell-shaped normal distribution envelope with most observations clustered around the mean and the remainder diminishing in number towards the tails.
Finally, a problem unique to large datasets is that the denominator in the t-test equation becomes diminishingly small as the number of daily samples increase. Consequently, the t‑statistic becomes exponentially large, together with the likelihood of finding significant differences that are too small to be meaningful. In statistical parlance this is known as Type1 error – the fallacy of declaring significance for differences that do not matter. Such differences could be due to single aberrations or outliers for instance.
A protocol
Using a parallel dataset related to a site move at Townsville airport in December 1994, a protocol has been developed to assist avoiding pitfalls in applying t-tests to timeseries of parallel data. At the outset, an estimate of effect size, determined as the raw data difference divided by the standard deviation (Cohens d) assesses if the difference between instruments/sites is likely to be meaningful. An excel workbook was provided with step-by-step instructions for calculating day-of-year (1-366) averages that define the annual cycle, constructing a look-up table and deducting respective values from data thereby producing de-seasoned anomalies. Anomalies are differenced as an additional variable (Site2 minus Site1, which is the control).
Having prepared the data, graphical analysis of their properties, including autocorrelation function (ACF) plots, daily data distributions, probability density function (PDF) plots, and inspection of anomaly differences assist in determining which data to compare (raw data or anomaly data). The dataset that most closely matches the underlying assumptions of independence and normality should be chosen and where autocorrelation is unavoidable, randomised data subsets offer a way forward. (Randomisation may be done in Excel and subsets of increasing size used in the analysis.)
Most analyses can be undertaken using the freely available statistical application PAST from the University of Oslo: https://www.nhm.uio.no/english/research/resources/past/ Specific stages of the analysis have been referenced to pages in the PAST manual.
The Brisbane Study
The Brisbane study replicates the previous Townsville study, with the aim of showing that protocols are robust. While the Townsville study compared thermometer and automatic weather station maxima measured in 60-litre screens located 172m apart, the Brisbane study compared Tmax for two AWS each with 60-litre screens, 3.2 km apart, increasing the likelihood that site-related differences would be significant.
While the effect size for Brisbane was triflingly small (Cohens d = 0.07), and the difference between data-pairs stabilised at about 940 sub-samples, a significant difference between sites of 0.25oC was found when the number of random sample-pairs exceeded about 1,600. Illustrating the statistical fallacy of excessive sample numbers, differences became significant because the dominator in the test equation (the pooled standard error) declined as sample size increased, not because the difference widened. PDF plots suggested it was not until the effect size exceeded 0.2, that simulated distributions showed a clear separation such that the difference between Series1 and Series2 of 0.62oC could be regarded as both significant and meaningful in the overall scheme of things.
Importantly, the trade-off between significance and effect size is central to avoiding the trap of drawing conclusions based on statistical tests alone.
Dr Bill Johnston
4 June 2023
Two important links – find out more
First Link: The page you have just read is the basic cover story for the full paper. If you are stimulated to find out more, please link through to the full paper – a scientific Report in downloadable pdf format. This Report contains far more detail including photographs, diagrams, graphs and data and will make compelling reading for those truly interested in the issue.
Click here to access a full pdf report containing detailed analysis and graphs
Second Link: This link will take you to a downloadable Excel spreadsheet containing a vast number of data used in researching this paper. The data supports the Full Report.
Click here to download a full Excel data pack containing the data used in this research
Why statistical tests matter
Fake-news, flash-bangs
and why statistical tests matter
Dr Bill Johnston
Main Points
Comparing instruments using paired t-tests, verses unpaired tests on daily data is inappropriate. Failing to verify assumptions, particularly that data are independent (not autocorrelated), and not considering the effect of sample size on significance levels creates illusions that differences between instruments are significant or highly significant when they are not. Using the wrong test and naïvely or bullishly disregarding test assumptions plays to tribalism not trust.
Investigators must justify the tests they use, validate that assumptions are not violated, that differences are meaningful and thereby show their conclusions are sound.
Discussion
Paired or repeated-measures t-tests are commonly used to determine the effect of an intervention by observing the same subjects before and after (e.g., 10 subjects before and after a treatment). As within-subjects variation is controlled, differences are attributable to the treatment. In contrast, un-paired or independent t‑tests compare the means of two groups of subjects, each having received one of two interventions (10 subjects that received one or no treatment vs. 10 that were treated). As variation between subjects contributes variation to the response, un-paired t-tests are less sensitive than paired tests.
Extended to a timeseries of sequential observations by different instruments (Figure 1), the paired t-test evaluates the probability that the mean of the difference between data-pairs (calculated as the target series minus the control) is zero. If the t‑statistic indicates the mean of the differences is not zero, the alternative hypothesis that the two instruments are different prevails. In this usage, significant means there is a low likelihood, typically less than 0.05, 5% or one in 20, that the mean of the difference equals zero. Should the P-value be less than 0.01, 0.001, or smaller, the difference is regarded as highly significant. Importantly, significant and highly significant are statistical terms that reflect the probability of an effect, not whether the size of an effect is meaningful.
To reiterate, paired tests compare the mean of the difference between instruments with zero, while un-paired t‑tests evaluate whether Tmax measured by each instrument is the same.
While sounding pedantic, the two tests applied to the same data result in strikingly different outcomes, with the paired test more likely to show significance. Close attention to detail and applying the right test is therefore vitally important.
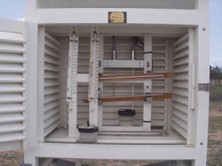
Figure 1. Inside the current 60-litre Stevenson screen at Townsville airport. At the front are dry and wet-bulb thermometers, behind are maximum (mercury) and minimum (alcohol) thermometers, held horizontally to minimise “wind-shake” which can cause them to re-set, and at the rear, which faces north, are dry and wet-bub AWS sensors. Cooled by a small patch of muslin tied by a cotton wick that dips into the water reservoir, wet-bulb depression is used to estimate relative humidity and dew point temperature. (BoM photograph).
Thermometers Vs PRT Probes
Comparisons of thermometers and PRT probes co-located in the same screen, or in different screens, rely on the air being measured each day as the test or control variable, thereby presuming that differences are attributable to instruments. However, visualize conditions in a laboratory verses those in a screen where the response medium is constantly circulating and changing throughout the day at different rates. While differences in the lab are strictly attributable, in a screen, a portion of the instrument response is due to the air being monitored. As shown in Figure 1, instruments that are not accessed each day are more conveniently located behind those that are, thereby resulting in spatial bias. The paired t-test, which apportions all variation to instruments is the wrong test under the circumstances.
Test assumptions are important
The validity of statistical tests depends on assumptions, the most important of which for paired t-tests is that differences at one time are not influenced by differences at previous times. Similarly for unpaired tests where observations within groups cannot be correlated to those previous. Although data should ideally be distributed within a bell-shaped normal-distribution envelope, normality is less important if data are random and numbers of paired observations exceed about 60. Serial dependence or autocorrelation reduces the denominator in the t-test equation, which increases the likelihood of significant outcomes (false positives) and fatally compromises the test.
Primarily caused by seasonal cycles the appropriate adjustment for daily timeseries is to deduct day-of-year averages from respective day-of-year data and conduct the right test on seasonally adjusted anomalies.
Covariables on which the response variable depends are also problematic. These includes heating of the landscape over previous days to weeks, and the effects of rainfall and evaporation that may linger for months and seasons. Removing cycles, understanding the data, using sampling strategies and P-level adjustments so outcomes are not biased may offer solutions.
Significance of differences vs. meaningful differences
A problem of using t-tests on long time series is that as numbers of data-pairs increase, the denominator in the t-test equation, which measures variation in the data, becomes increasingly small. Thus, the ratio of signal (the instrument difference) to noise (the standard error, pooled in the case of un-paired tests) increases. The t‑value consequently becomes exponentially large, the P-level declines to the millionth decimal place and the test finds trifling differences to be highly significant, when they are not meaningful. So, the significance level needs to be considered relative to the size of the effect.
For instance, a highly significant difference that is less than the uncertainty of comparing two observations (±0.6oC) could be an aberration caused by averaging beyond the precision of the experiment (i.e., averaging imprecise data to two, three or more decimal places).
The ratio of the difference to the average variation in the data [i.e., (PRTaverage minus thermometeraverage) divided by the average standard deviation], which is known as Cohens d, or the effect size, also provides a first-cut empirical measure that can be calculated from data summaries to guide subsequent analysis.
Cohens d indicates whether a difference is likely to be negligible (less than 0.2 SD units), small (>0.2), medium (>0.5) or large (<0.8), which identifies traps to avoid, particularly the trap of unduly weighting significance levels that are unimportant in the overall scheme of things.
The Townsville case study
T-tests of raw data were invalidated by autocorrelation while those involving seasonally adjusted anomalies showed no difference. Randomly sampled raw data showed significance levels depended on sample size not the difference itself, thus exposing the fallacy of using t‑tests on excessively large numbers of data-pairs. Irrespective of the tests, the effect size calculated from the data summary of 0.12 SD units is trivial and not important.
Conclusions
Using paired verse unpaired t-tests on timeseries of daily data inappropriately, not verifying assumptions, and not assessing the effect size of the outcome creates division and undermines trust. As illustrated by Townsville, it also distracts from real issues. Using the wrong test and naïvely or bullishly disregarding test assumptions plays to tribalism not trust.
A protocol is advanced whereby autocorrelation and effect size are examined at the outset. It is imperative that this be carried out before undertaking t-tests of daily temperatures measured in-parallel by different instruments.
The overarching fatal error is using invalid tests to create headlines and ruckus about thin-things that make no difference, while ignoring thick-things that would impact markedly on the global warming debate.
Two important links – find out more
First Link: The page you have just read is the basic cover story for the full paper. If you are stimulated to find out more, please link through to the full paper – a scientific Report in downloadable pdf format. This Report contains far more detail including photographs, diagrams, graphs and data and will make compelling reading for those truly interested in the issue.
Click here to download the full paper Statistical_Tests_TownsvilleCaseStudy_03June23
Second Link: This link will take you to a downloadable Excel spreadsheet containing a vast number of data points related to the Townsville Case Study and which were used in the analysis of the Full Report.
Click here to access the full data used in this post Statistical tests Townsville_DataPackage
Halls Creek, Western Australia
Is homogenisation of Australian temperature data any good?
Dr Bill Johnston[1]
Background
Homogenisation of Australian temperature data commenced in the late 1980s and by 1996 under the watchful eye of Bureau of Meteorology (BoM) scientist Neville Nicholls, who at that time was heavily involved with the World Meteorological Organisation (WMO) and the fledgling Intergovernmental Panel on Climate Change (IPCC), the first Australian high-quality homogenised temperature dataset (HQ1) was produced by Simon Torok. This was followed in succession by an updated version in 2004 (HQ2) that finished in 2011, then the Australian Climate Observations Reference Network – Surface Air Temperature (ACORN-SAT) dataset, with version 1 (AcV1) released in 2012 being updated until 2017. AcV2 replaced ACV1 from 2018, with the most recent iteration AcV2.3 updated to December 2021.
Why is homogenisation important?
Data homogenisation represents the pinnacle of policy-driven science, meaning that following release of the First Assessment Report by the Intergovernmental Panel on Climate Change (IPCC) in 1990, for which Neville Nicholls was a substantial contributor, the Australian government set in-place a ‘climate’ agenda. Although initially rejected by Cabinet, in 1989 Labor Senator Graham Richardson proposed a 20% reduction in 1988 Australian greenhouse gas emission levels by 2005. The target was adopted in October 1990 as a bipartisan policy (i.e., by both major Australian political parties) and endorsed by a special premiers conference in Brisbane as the InterGovernmental Agreement on the Environment in February 1992 (https://faolex.fao.org/docs/pdf/aus13006.pdf). Following that meeting in February, the Council of Australian Governments (COAG) was set-up by Labor Prime Minister Paul Keating in December 1992.
As outlined by the Parliamentary Library Service: https://www.aph.gov.au/About_Parliament/ Parliamentary_Departments/Parliamentary_Library/pubs/rp/rp1516/Climate2015 this was the mechanism whereby the most important and far-reaching policy agenda since Federation in 1901, was ushered into place without a vote being cast by the unsuspecting electorate. However, in order to support the policy:
- Land-surface temperatures had to be shown to be warming year-on-year, particularly since 1950.
- Models were needed that predicted climate calamities into the future.
- Natural resources -related science, which was previously the prerogative of the States, required reorganisation under a funding model that guided outcomes in the direction of the policy agenda.
- Particular attention was also paid to messaging climate alarm regularly and insistently by all levels of government.
As it provides the most tangible evidence of climate warming, trend in maximum temperature (Tmax) is of overarching importance. It is also the weakest link in the chain that binds Australians of every creed and occupation to the tyranny of climate action. If homogenisation of Tmax data is unequivocally shown to be a sham, other elements of the policy, including evidence relied on by the IPCC are on shaky ground. This is the subject of the most recent series of reports published by www.bomwatch.com.au.
The question in this paper is whether trend and changes in the combined Tmax dataset for Halls Creek, reflect site and instrument changes or changes in weather and climate.
Halls Creek maximum temperature data
Detailed analyses of Halls Creek Tmax using objective, replicable physically-based BomWatch protocols found data were affected by a change at the old post office in 1917, another in 1952 after the site moved to the Aeradio office at the airport in 1950, and another in 2013 due to houses being built within 30m of the Stevenson screen two years before it relocated about 500m southeast to its present position in September 2015. Three step-changes resulted in four data segments; however, mean Tmax for the first and third segments were not different.
While the quality of data observed at the old post office was inferior to that of sites at the airport, taking site changes and rainfall into account simultaneously left no trend or change in Tmax data that could be attributed to climate change, CO2, coal mining, electricity generation or anything else. Furthermore, step-changes in the ratio of counts of data less than the 5th and greater than the 95th day-of-year dataset percentiles (low and high extremes respectively) were attributable to site changes and not the climate. Nothing in the data therefore suggests the climate of the region typified by Halls Creek Tmax has warmed or changed.
Homogenisation of Halls Creek data
As it was originally conceived, homogenisation aimed to remove the effects non-climate impacts on data, chief amongst those being weather station relocations and instrument changes, so homogenised data reflected trends and changes in the climate alone. In addition, ACORN-SAT sought to align extremes of data distributions so, in the words of Blair Trewin, data “would be more homogeneous for extremes as well as for means”. To achieve this, Trewin used first-differenced correlated reference series and complex methods to skew data distributions at identified changepoints based on transfer functions. This was found to result in an unrealistic exponential increase in upper-range extremes since 1985.
Reanalysis using BomWatch protocols and post hoc tests and scatterplots showed that in order to achieve statistically significant trends, homogenisation cooled past temperatures unrealistically and too aggressively. For instance, cool-bias increased as observed Tmax increased. It was also found that the First Law of Thermodynamics on which BomWatch protocols are based, did not apply to homogenised Tmax data. This shows that the Bureau’s homogenisation methods produce trends in homogenised Tmax data that are unrelated to the weather, and therefore cannot reflect the climate.
As it was consensus-driven and designed to serve the political ends of WWF and Australia’s climate industrial elites, and it has no statistical, scientific or climatological merit, the ACORN-SAT project is a disgrace and should be abandoned in its entirety.
[1] Former NSW Department of Natural Resources research scientist and weather observer.
Two important links – find out more
First Link: The page you have just read is the basic cover story for the full paper. If you are stimulated to find out more, please link through to the full paper – a scientific Report in downloadable pdf format. This Report contains far more detail including photographs, diagrams, graphs and data and will make compelling reading for those truly interested in the issue.
Click here to download the full paper with photos graphs and data.
Second Link: This link will take you to a downloadable Excel spreadsheet containing a vast number of supporting data points for the Potshot (Learmonth) paper.
Learmonth-Potshot-&-ACORN-SAT
Is homogenisation of Australian temperature data any good?
Part 5. Potshot and ACORN-SAT
Dr Bill Johnston
Former NSW Department of Natural Resources research scientist and weather observer.
Careful analysis using BomWatch protocols showed that ACORN-SAT failed in their aim to “produce a dataset which is more homogeneous for extremes as well as for means”. Their failure to adjust the data for a step-change in 2002 shows unequivocally that methodology developed by Blair Trewin lacks rigour, is unscientific and should be abandoned.
Read on …
Potshot was a top-secret long-shot – a WWII collaboration between the United States Navy, the Royal Australian Air Force and Australian Army, that aimed to deter invasion along the lightly defended north-west coast of Western Australia and take the fight to the home islands of Japan. It was also the staging point for the 27- to 33-hour Double-Sunrise Catalina flying-boat service to Ceylon (now Sri Lanka) that was vital for maintaining contact with London during the dark years of WWII. It was also the base for Operation Jaywick, the daring commando raid on Singapore Harbour by Z-Force commandos in September 1943.
The key element of Potshot was the stationing of USS Pelias in the Exmouth Gulf to provide sustainment to US submarines operating in waters to the north and west. To provide protection, the RAAF established No. 76 OBU (Operational Base Unit) at Potshot in 1943/44 and, at the conclusion of hostilities, OBU Potshot was developed as RAAF Base Learmonth, a ‘bare-base’ that can be activated as needed on short-notice. Meteorological observations commenced at the met-office in 1975. Learmonth is one of the 112 ACORN-SAT sites (Australian Climate Observations Reference Network – Surface Air Temperature) used to monitor Australia’s warming. Importantly, it is one of only three sites in the ACORN-SAT network where data has not been homogenised.
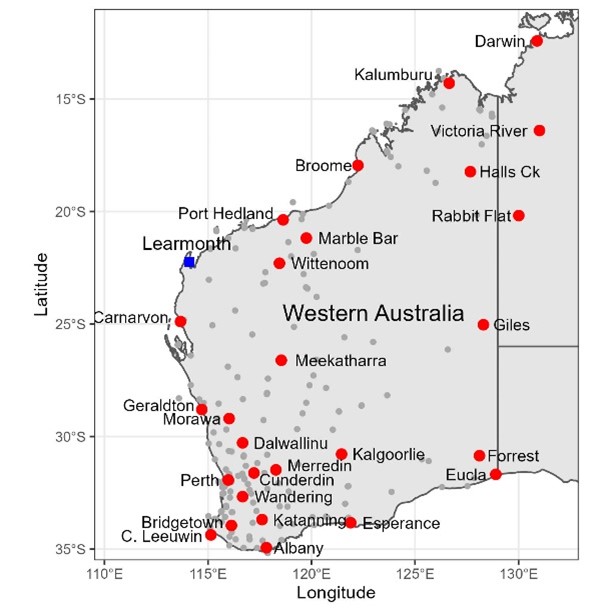
Potshot was the top-secret WWII base that transitioned to RAAF Base Learmonth at the conclusion of WWII.
- By not adjusting for the highly significant maximum temperature (Tmax) step-change in 2002 detected by BomWatch, ACORN-SAT failed its primary objective which is to “produce a dataset which is more homogeneous for extremes as well as for means”.
- Either Blair Trewin assumed the 12-year overlap would be sufficient to hide the effect of transitioning from the former 230-litre Stevenson screen to the current 60-litre one; or his statistical methods that relied on reference series were incapable of objectively detecting and adjusting changes in the data.
- In either case it is another body-blow to Trewin’s homogenisation approach. Conflating the up-step in Tmax caused by the automatic weather station and 60-litre screen with “the climate” and lack of validation within the ACORN-SAT project generally, unethically undermines the science on which global warming depends.
- Due to their much-reduced size, and lack of internal buffering, 60-litre Stevenson screens are especially sensitive to warm eddies that arise from surfaces, buildings etc. that are not representative of the airmass being monitored.
- Increased numbers of daily observations/year ≥95th day-of-year dataset percentiles relative to those ≤5th day-of-year percentiles, which explains the up-step, is a measurement issue, not a climatological one.
By omission in the case of Learmonth, as ACORN-SAT produces trends and changes in homogenised data that do not reflect the climate, the project and its peers including others run under the guise of the World Meteorological Organisation of which Trewin is a major player, should be abandoned.
Dr. Bill Johnston
12 January 2023
Two important links – find out more
First Link: The page you have just read is the basic cover story for the full paper. If you are stimulated to find out more, please link through to the full paper – a scientific Report in downloadable pdf format. This Report contains far more detail including photographs, diagrams, graphs and data and will make compelling reading for those truly interested in the issue.
Click here to download the full paper with photos graphs and data.
Second Link: This link will take you to a downloadable Excel spreadsheet containing a vast number of supporting data points for the Carnarvon paper.
Click here to download the Excel spreadsheet
Postscript
Dr Trewin and his peers, including those who verify models using ACORN-SAT data, scientists at the University of NSW including Sarah Perkins-Kilpatrick, those who subscribe to The Conversation or The Climate Council are welcome to fact-check or debate the outcome of our research in the open by commenting at www.bomwatch.com.au. The Datapack relating to the Potshot Report is available here Learmonth_DataPack
Carnarvon, Western Australia
Is homogenisation of Australian temperature data any good?
Part 4. Carnarvon, Western Australia
Dr Bill Johnston[1]
scientist@bomwatch.com.au
The ACORN-SAT project is deeply flawed, unscientific and should be abandoned.
Read on …
Maximum temperature data for Carnarvon, Western Australia is surprisingly no use at all for tracking climatic trend and change. With Learmonth close second at Longitude 114.0967o, Carnarvon (113.6700o) is the western-most of the 112 stations that comprise the ACORN-SAT network (Australian Climate Observations Reference Network – Surface Air Temperature) used to monitor Australia’s warming (Figure 1). Carnarvon is also just 1.4o Latitude south of the Tropic of Capricorn but unlike Learmonth, which receives moderate rain in February, Carnarvon receives practically none from the end of August through to March. Due to its vast underground aquifer, the lower Gascoyne is the most productive irrigation area in WA and most of the vegetables, melons and fruits sold in Perth are grown around Carnarvon.
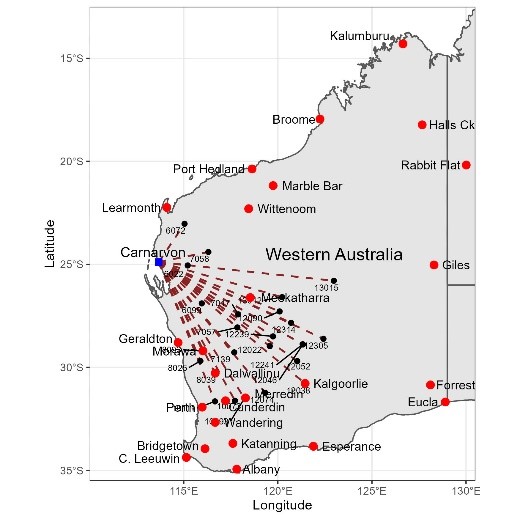
Figure 1. The distribution of ACORN-SAT sites in WA, as well as all the neighbouring sites used by ACORN-SAT v.3 to homogenise Carnarvon data.
Located about 900 km north of Perth, Carnarvon post office, built in 1882, was an important link in the expanding WA telegraph network and the aerodrome was an important refuelling stop. Runways were lengthened by the Royal Australian Air Force and during WWII it was used as a forward operation base
Servicing the main 1930s west-coast air corridor from Perth to Darwin, and Java and on to Europe, Aeradio was established by the Air Board in Geraldton, Carnarvon, Port Hedland, and at aerodromes every 300 km or so further north, and around Australia in 1939/40. A close-up view of Carnarvon Aeradio snipped from a 1947 aerial photograph is shown in Figure 2. While weather observers trained by the Bureau of Meteorology (BoM) in Melbourne undertook regular weather observations, prepared forecasts and provided pilot briefings, radio operators maintained contact with aircraft and advised of inclement conditions. As units were well-distributed across the continent, combined with post officers that reported weather observations by telegraph, the aeradio network formed the backbone of ACORN-SAT. Homogenisation of their data with that of post offices, lighthouses and pilot stations ultimately determines the apparent rate of warming in Australia’s climate.
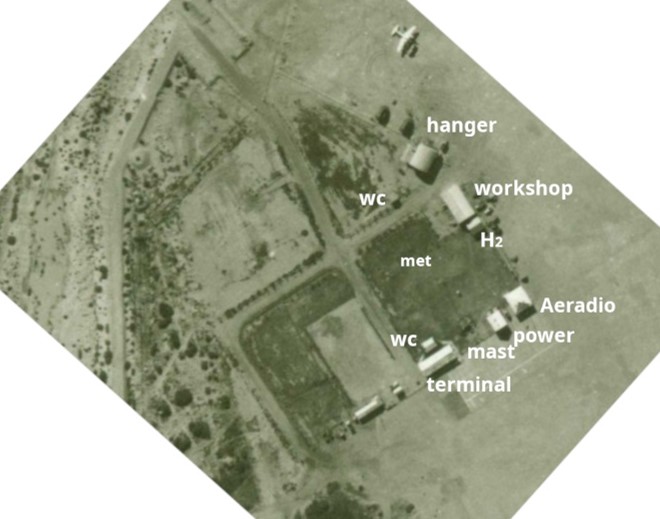
Figure 2. A snip of the Carnarvon aerodrome operations precinct in 1949 showing location of facilities and that are area in the vicinity of the met-enclosure (met) was watered. (Three additional radio masts were located within in the watered area and watering was probably necessary to ensure grounding of the earth-mat buried in the dry soil.)
Despite several rounds of peer review the question of whether trends and changes in raw data are homogenised Tmax data reflect site and changes or the true climate has not been independently assessed.
The research is important. Alteration of data under the guise of data homogenisation, flows through to BoM’s annual climate statements, CSIRO’s State of the Environment, and State of the Climate reports, reports put out by IPCC, COP, and the World Economic Forum, and ultimately government supported scare campaigns run by WWF, the Climate Council and other green groups, that underpin the unattainable goal of net-zero.
Commencing around 1990, Australia’s homogenisation campaign has been overseen by Dr Neville Nicholls, Emeritus Professor at Monash University, a loud and vocal supporter of the warming hypotheses. Changing the data on which it is based, then claiming in collegially peer-reviewed publications that the climate is warming, risks considerable economic, strategic and political harm. Australia is being weakened from within by Nicholls and his protégés, including those within CSIRO, and every aspect of the economy, from de-industrialisation, to attacks on agriculture, to looming conflict with China is predicated by temperature data homogenisation.
Summary findings
- Good site control is fundamental to any experiment investigating long-term trend and change. However, the site at the post office was shaded, watered and generally poor, the Aeradio site was also watered, while the site at the meteorological office was subject to multiple changes after 1990.
- Homogenisation failed to undertake basic quality assurance and frequency analysis, so could not objectively adjust for the effect of extraneous factors such as watering, site chances etc. Consequently, as ACORN-SAT data for Carnarvon were not homogeneous either for extremes or annual means it failed its primary objective.
While changing data to agree with hypotheses is unscientific and dishonest, the most obvious homogenisation subterfuge is the adjustment of changes that made no difference to the data, while ignoring those that did. Second, using reference series comprised of neighbouring datasets without ensuring they are homogeneous.
The use of correlated data that likely embed parallel faults to disproportionally correct faults in ACORN-SAT data and thereby embed trends in homogenised data, has no statistical or scientific merit. As the ACORN-SAT project is misleading irredeemably deeply flawed and is a clear danger to Australia’s prosperity and security, it should be abandoned.
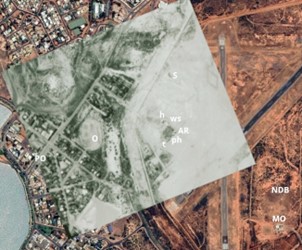
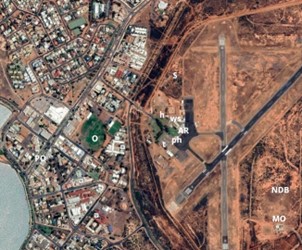
A reoriented and rescaled overlay of an aerial photograph showing the watered airport precinct relative to the location of the post office in 1947, and the November 2021 Google Earth Pro satellite image, locating the same sites. Runways were unsealed in 1947 and there were still several splinter-proof aircraft shelters visible (marked ‘s’). By 2021 they had moved the non-directional beacon (NDB) from behind the power house (ph), and the site to the right of the meteorological office (MO) had been moved out of the way of the access road. The MO closed in 2016.
3 January 2023
Two important links – find out more
First Link: The page you have just read is the basic cover story for the full paper. If you are stimulated to find out more, please link through to the full paper – a scientific Report in downloadable pdf format. This Report contains far more detail including photographs, diagrams, graphs and data and will make compelling reading for those truly interested in the issue.
Click here to download the full paper with photos graphs and data
Second Link: This link will take you to a downloadable Excel spreadsheet containing a vast number of supporting data points for the Carnarvon paper.
Click here to download the Excel spreadsheet for Carnarvon
Note: Line numbers are provided in the linked Report for the convenience of fact checkers and others wishing to provide comment. If these comments are of a highly technical nature, relating to precise Bomwatch protocols and statistical procedures, it is requested that you email Dr Bill Johnston directly at scientist@bomwatch.com.au referring to the line number relevant to your comment.
[1] Former NSW Department of Natural Resources research scientist and weather observer.